In the ever-evolving realm of data and technology, understanding the knowledge graph definition becomes crucial. As industries progressively embrace advanced technologies, a recurring question consistently arises: how can we navigate the vast web of interconnected data? Interestingly, the solution lies in the powerful knowledge graph definition.
Often regarded as the backbone of intelligent systems, knowledge graphs empower various tech tools, from search engines to virtual assistants. Furthermore, by offering a unique visualization method for vast data, they allow for the extraction of valuable connections and insights.
We’ve already written about why knowledge graphs are superior to chatbots.
Now, one might wonder, what exactly does the term "knowledge graph definition" entail?
Diving deeper, in this article, we'll explore the knowledge graph definition, its benefits, challenges, and its pivotal role in contemporary data management.
Knowledge Graph Definition: An In-depth Look
At its essence, a knowledge graph is a structured method of representing and linking data. Let's dissect this knowledge graph definition further.
Key Elements of a Knowledge Graph Definition
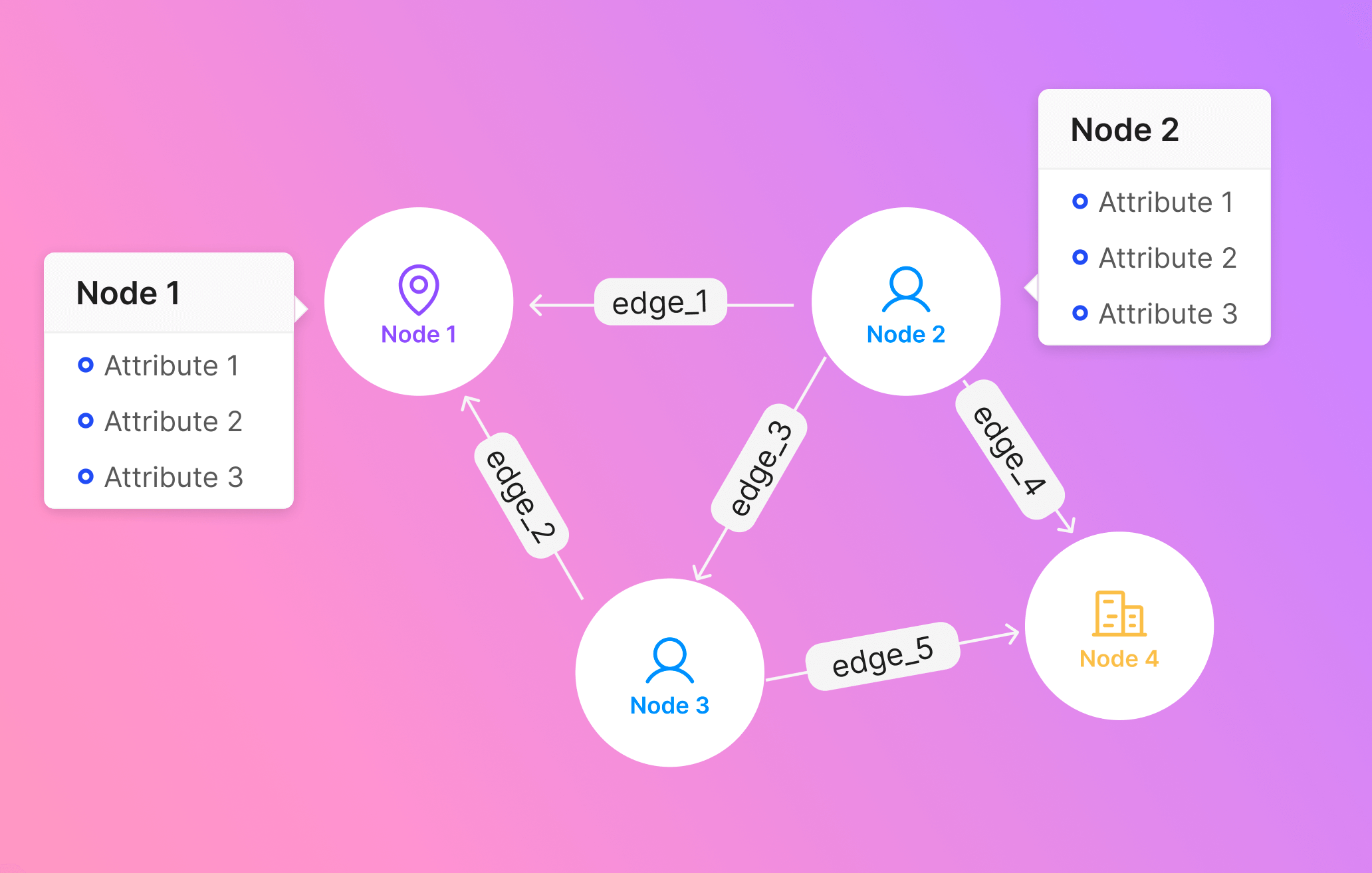
- Nodes: At the heart of our knowledge graph definition are nodes, the primary entities or data points in the graph. In a literature-based database, nodes might signify authors, titles, publishers, and genres.
- Edges: These are the connectors in the graph, symbolizing the relationships between nodes. An edge might, for instance, associate an author with a book they've penned or a book with its respective genre.
- Attributes: Further enriching the knowledge graph definition are attributes that offer supplementary details about nodes. For instance, a book's publication year or its number of pages.
Visualizing a Knowledge Graph: The Essence of its Definition
..png)
A knowledge graph is akin to a vast web or network. Within this construct, individual points or dots stand as nodes (specific data points), and the ties between them are edges (the relationships).
Observing this structure reveals the intricate patterns of how data pieces correlate and interact, as illustrated above.
How Knowledge Graphs Revolutionize Traditional Databases
In the realm of data storage, traditional databases typically store data in fixed tables and columns. However, in contrast to this, the knowledge graph definition emphasizes the profound connections between data segments. This approach isn't just about passively storing data; rather, it's about actively understanding how one piece of information intricately relates to another, thereby providing context and deeper meaning.
Take, for example, a vector database. It might straightforwardly tell you that a person bought a book. On the other hand, when looking through the lens of a knowledge graph, you'd be privy to a broader picture: the other books by the same author that the person might have perused, the similar genres they have shown a preference for, or even suggestions about other authors they might find appealing based on their previous purchase history.
In essence, a knowledge graph doesn't just provide a myopic view; it offers a comprehensive, 360-degree panorama of data. By prioritizing connections and context over mere storage, it emerges as an invaluable tool, especially in today's information-saturated world.
Benefits Derived from the Knowledge Graph Definition
The knowledge graph definition is not just about connecting data points; it's about understanding the complex web of relationships and extracting value from it. Embodying a unique approach to data representation, knowledge graphs bring forth several unparalleled advantages:
1. Enhanced Data Visualization
- Simplified Complexity: Knowledge graphs morph intricate data ties into an intelligible visual format. By utilizing the principles from the knowledge graph definition, organizations can offer stakeholders unmatched clarity for decision-making.
- Interconnected Insights: The holistic view provided by knowledge graphs showcases connections that might otherwise remain obscured. By fully grasping our knowledge graph definition, one realizes the depth of insights that can be pulled from seemingly unrelated data points.
2. Comprehensive Data Interpretation
- Contextual Understanding: Beyond mere data points, knowledge graphs, as outlined in our knowledge graph definition, emphasize the underlying connections, enabling businesses to discern patterns and trends that traditional databases might miss.
- Deeper Data Exploration: Knowledge graphs enable users to dive deep into data points, revealing layers of connections and insights, ensuring that no piece of information exists in isolation.
3. Efficient Decision Making
- Informed Strategies: By offering a panoramic view of interrelated data, knowledge graphs, true to the knowledge graph definition, arm decision-makers with the context they need to formulate informed strategies.
- Predictive Capabilities: Harnessing the power of knowledge graphs allows businesses to anticipate market trends and user behaviors, positioning them a step ahead of the competition.
4. Personalized User Experiences
- Tailored Interactions: By understanding intricate user behaviors and preferences derived from the knowledge graph definition, platforms can deliver content, recommendations, or services that resonate with individual users.
- Enhanced Engagement Metrics: Personalized experiences, powered by knowledge graphs, result in increased user engagement, satisfaction, and loyalty, thus driving growth and reducing churn rates.
5. Seamless Data Integration
- Unified Data View: As the knowledge graph definition suggests, these graphs excel in amalgamating diverse data sources, providing businesses with a singular, cohesive view of their data landscape.
- Scalable Architectures: Knowledge graphs are inherently designed to grow and evolve. As organizations expand and data sources multiply, knowledge graphs ensure smooth integrations without compromising performance.
In essence, by adhering to the principles of the knowledge graph definition, businesses can elevate their data management strategies from mere storage solutions to dynamic systems that offer actionable insights, predictive analytics, and enhanced user experiences.
Challenges in Implementing the Knowledge Graph Definition
Implementing the principles outlined in the knowledge graph definition is a powerful step for any organization. However, truly harnessing its potential is not without its hurdles. To make the most of a knowledge graph, it's imperative to understand and anticipate these challenges:
Data Quality and Consistency
- The Importance of Accurate Data: A knowledge graph's strength lies in its data. If erroneous or outdated data is introduced, it can mislead and produce flawed insights, making adherence to the knowledge graph definition critical for maintaining quality.
- Uniformity Across the Board: With expanding data sources and evolving information, ensuring consistent representation in line with the knowledge graph definition can be daunting.
Technical Challenges and Maintenance
- Expertise Requirement: Implementing a knowledge graph, as described in the knowledge graph definition, demands technical proficiency. Some organizations might find this a barrier, especially when dealing with nuanced data interrelationships.
- Continuous Refinement: Just as data and insights evolve, knowledge graphs require ongoing updates. This means constant engagement and resources to ensure the graph aligns with the dynamic knowledge graph definition.

At Lettria, we aim to solve for this complexity in creation and maintenance. Our knowledge structuration tool is able to automatically generate and maintain knowledge graphs using raw, unstructured text as input.
If you’re interested in knowing more about our tool, check out our page on our text to graph data pipeline.
Scalability and Integration
- Vastness of Data: As organizations grow, so does their data. Ensuring that a knowledge graph can handle this exponential growth, while staying true to the knowledge graph definition, is essential.
- Smooth Incorporation: Every new data source or type presents a unique challenge. Adhering to the knowledge graph definition while integrating these seamlessly is a complex yet vital task.
Privacy and Ethical Considerations
- Protecting Sensitive Information: Knowledge graphs, as understood from the knowledge graph definition, interlink myriad data points. Some of these can be private or sensitive, making their protection paramount.
- Adhering to Regulations: Compliance with data regulations, like GDPR, is non-negotiable. Those looking to implement the knowledge graph definition should be wary of how they handle personal data within their graphs.
Overcoming Potential Bias
- Counteracting Existing Prejudices: A knowledge graph can inadvertently perpetuate biases present in its input data. Adhering strictly to the knowledge graph definition and ensuring data neutrality can help alleviate this concern.
- Need for Vigilant Evaluation: To prevent skewed insights, stakeholders must critically assess the outputs of their knowledge graphs, even if they align with the knowledge graph definition.
Financial Implications
- Initial Capital: Establishing a comprehensive knowledge graph can demand significant investment. Organizations must evaluate if the potential benefits align with the costs, especially when adhering to the knowledge graph definition.
- Ongoing Expenditures: Beyond the initial setup, the maintenance, updates, and refinements of a knowledge graph can have recurring costs. Balancing these with the value derived from the knowledge graph definition is essential for long-term sustainability.
Recognizing these challenges is the first step towards a successful knowledge graph implementation. With a clear understanding of both the benefits and potential pitfalls of the knowledge graph definition, organizations can navigate their data journey with clarity and purpose.
In Conclusion
In order to aptly navigate today's intricate data maze, we need tools that are both resilient and insightful. Enter the knowledge graph definition: it provides just that—a potent solution that marries visualization with context, delivering actionable insights.
Moreover, knowledge graphs are rewriting how businesses interpret and deploy data, sealing the gaps between isolated data chambers. However, while their potential is undeniably vast, it's imperative for ventures to pinpoint and tackle the associated challenges. By doing so, this ensures that they can harness knowledge graphs to their utmost, fostering data-led decisions, elevating user experiences, and securing a competitive edge.
Looking ahead, as the digital horizon expands, the role of knowledge graphs in shaping the future of data analytics and management will undoubtedly become even more central. Consequently, enterprises that embrace this tool, cognizant of its strengths and limitations, are poised to flourish in the forthcoming digital age.