Understanding GraphRAG
Lettria is taking retrieval-augmented generation (RAG) to the next level, merging graph technology with the standard vector approach to create something better. Our goal is to help enterprises benefit from RAG technology without the risks of hallucinations or black-box responses.

A new type of RAG: Merging knowledge graphs with vector databases
Goodbye, Hallucinations
Current large language models (LLMs) are held back by the limitations of vector databases. The most obvious of these limitations are seen when the machine produces “hallucinations”. To address this problem and improve LLM accuracy, the RAG approach has been helpful, but not sufficient given the drawbacks of vectors.
That’s why Lettria has developed Knowledge Graphs, providing more context and accuracy in order to stop hallucinations and restore trust in generative AI.
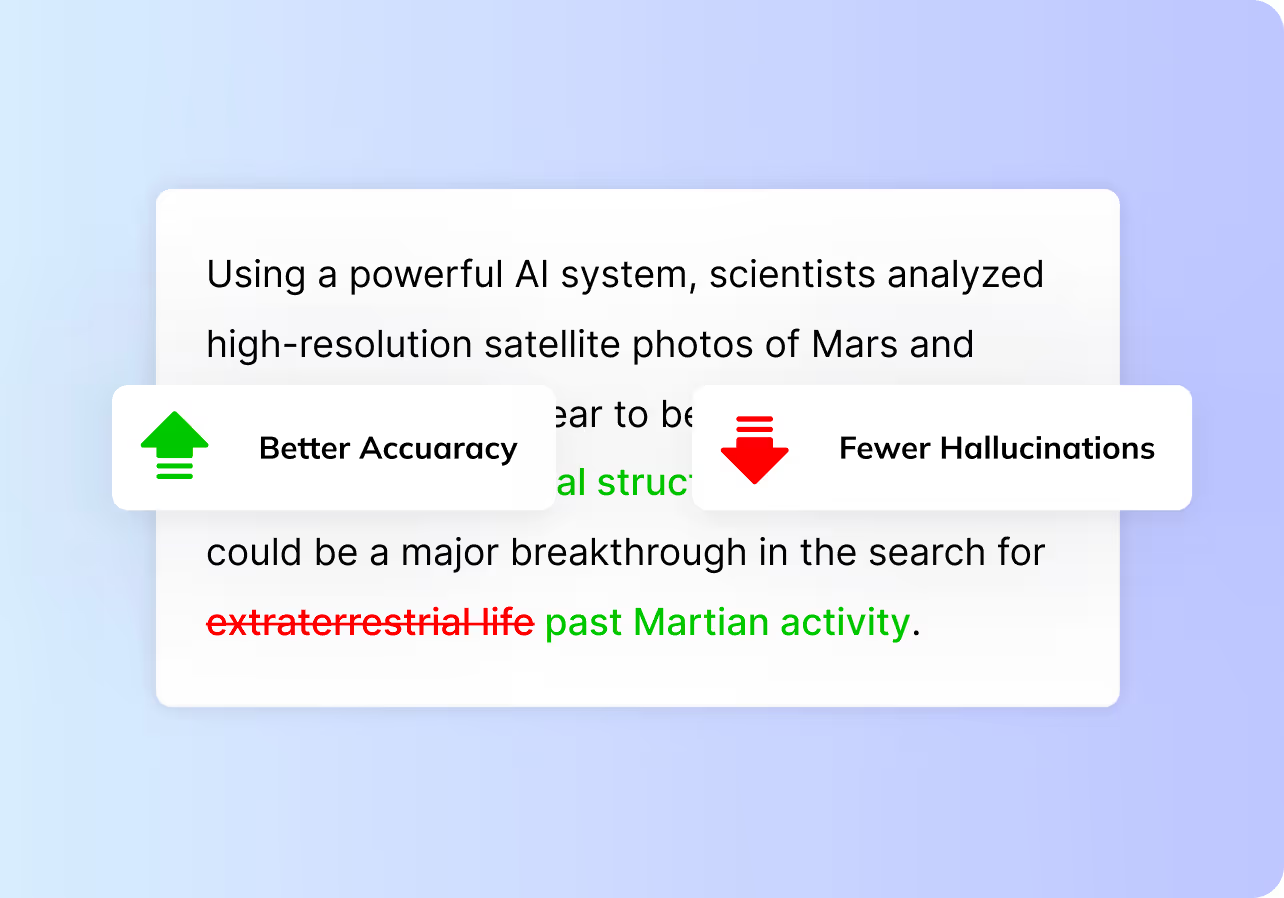
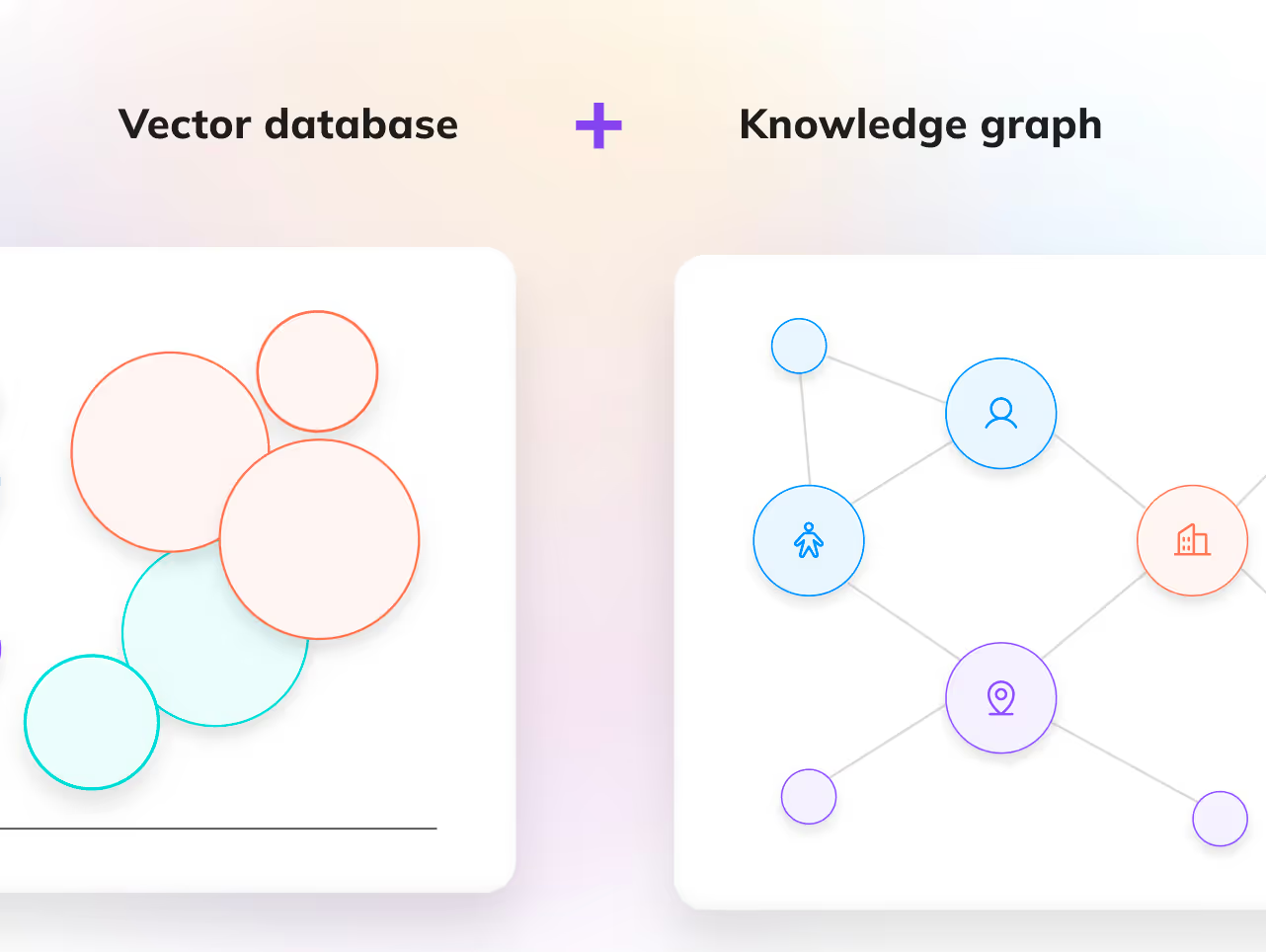
The best of both worlds
At Lettria, we’re delivering a better AI future thanks to the hybridization of the two RAG worlds. The result is faster, more accurate, and more contextually-aware AI.
Vectors provide a fast, efficient filtering system that narrows down the search space. Knowledge graphs then step in to understand relationships and offer rich, traceable context.
Hello, GraphRAG
Lettria has built its Knowledge Studio on the GraphRAG approach. By merging the contextual richness of knowledge graphs with the dynamic power of RAG tasks, we provide the environment that LLMs need to more accurately answer the pressing questions in your company.
The result? Precise, relevant, and insightful answers that capture the true essence of your business knowledge.

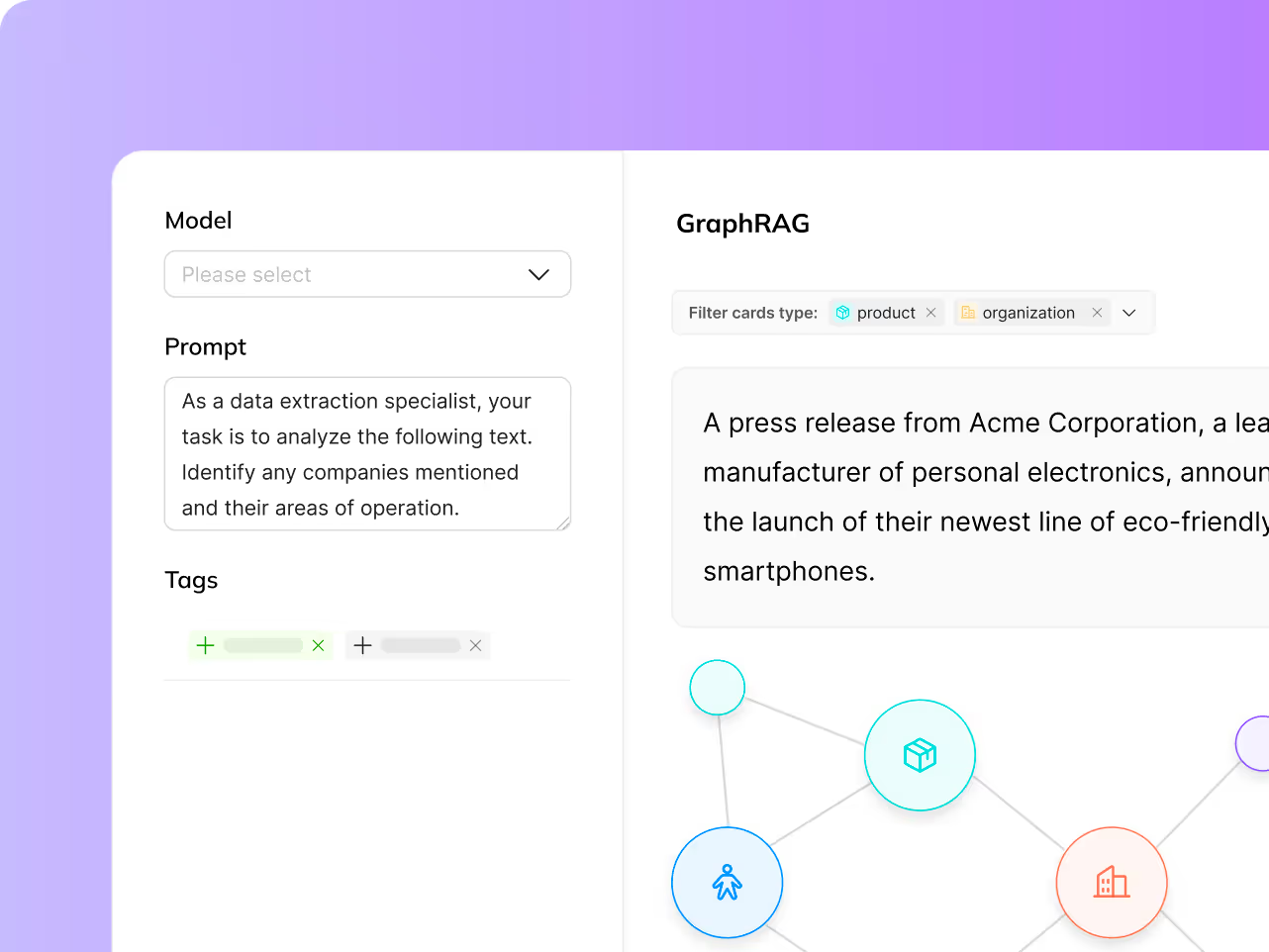
Improved accuracy, performance and scalability
With GraphRAG, the idea of “chatting with your data” becomes a reality, as data is transformed from a store of knowledge to an active partner.
Your unstructured data becomes usable and useful, ready to respond to day-to-day needs throughout your business.
How Lettria does it
Your texts, documents, and data – structured and unstructured – are turned into a graph.
1. Document importation and parsing
Each data source is carefully cleaned and preprocessed in order to extract text chunks and store metadata.
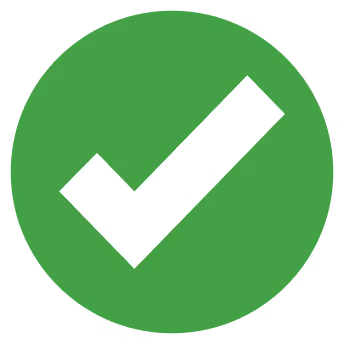
Entity recognition and linking Recognition and Linking
The chunks are processed through Lettria’s natural language structuration API, identifying entities and relationships between them in order to produce a knowledge graph.
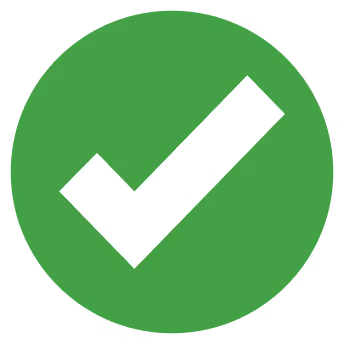
3. Embeddings and vector management
In parallel, the chunks are vectorized in order to maintain performance.
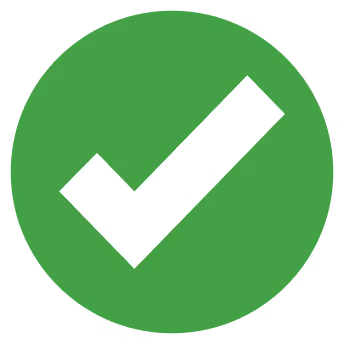
4. Database merging and reconciliation
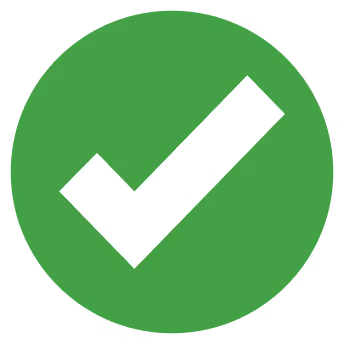
The structured output from our API and the embeddings are stored in a single database, ready to power all of your RAG needs.
Frequently Asked Questions
Currently, Lettria operates on a demo-based access model. To explore GraphRAG's capabilities and see how it can benefit your organization, you can request a personalized demo through the website.
No, Lettria's platform is designed to be user-friendly, allowing users without technical backgrounds to leverage GraphRAG's capabilities effectively.
GraphRAG processes data within a secure environment, ensuring that sensitive information remains confidential and is accessible only to authorized users within your organization.
Yes, GraphRAG is equipped to process complex data structures, including tables. It can convert tabular data into graph formats, enabling more effective querying and analysis.
GraphRAG is versatile and can be applied across various industries, including:
- Healthcare: Structuring patient data for better insights.
- Finance: Enhancing analysis of financial documents.
- Legal: Improving the organization and retrieval of legal texts.
- Engineering: Managing complex technical documentation.
The transformation process involves:
- Document Importation and Parsing: Uploading and preprocessing documents to extract text chunks and metadata.
- Entity Recognition and Linking: Identifying entities and their relationships to construct a knowledge graph.
- Embeddings and Vector Management: Vectorizing text chunks to facilitate efficient retrieval.
- Database Merging and Reconciliation: Combining structured outputs and embeddings into a unified database for RAG applications.
Traditional RAG models rely solely on vector embeddings, which can lead to issues like hallucinations due to a lack of contextual understanding. GraphRAG addresses this by integrating knowledge graphs, enabling the system to comprehend relationships between entities, thereby reducing inaccuracies and improving the relevance of generated content.
Lettria's GraphRAG is an advanced Retrieval-Augmented Generation (RAG) solution that combines the speed of vector-based search with the contextual depth of knowledge graphs. This hybrid approach enhances the accuracy and reliability of AI-generated responses by providing structured context alongside traditional vector embeddings.
Read more about GraphRAG
%20(1).png)
Patrick Duvaut
Head of Innovation
%20(1).png)
Patrick Duvaut
Head of Innovation
%20(1).png)
Patrick Duvaut
Head of Innovation